Essential guide to financial forecasts
Forecasts. If you’re a meteorologist, you can get your predictions wrong with some regularity, but most people will forget that you missed the mark and hope for a sunnier day. If you’re in finance, you can’t get it wrong. Your business depends on accurate financial forecasts and hope is not a strategy.
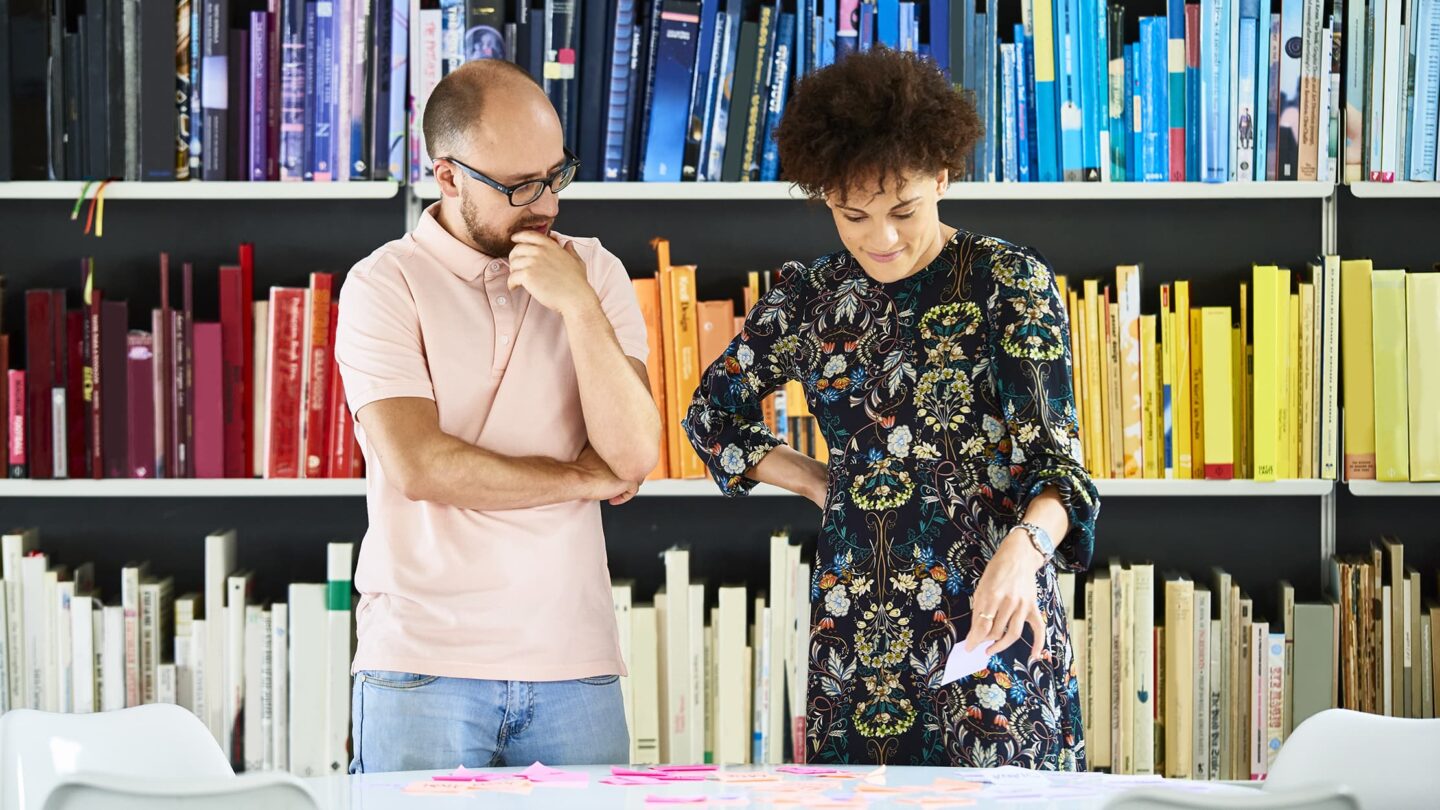
June 20, 2023 9 min read Leave a comment
Share Copy Link Copied
Forecasts. If you’re a meteorologist, you can get your predictions wrong with some regularity, but most people will forget that you missed the mark and hope for a sunnier day. If you’re in finance, you can’t get it wrong. Your business depends on accurate financial forecasts and hope is not a strategy.
Financial forecasting is the process of making predictions about a company’s financial performance, typically for future periods. It involves analyzing historical financial data and trends, as well as other relevant information, such as economic conditions, industry trends and market factors. Financial forecasting is critical for budgeting, strategic planning and investment decision-making.
There are many different methods and techniques that can be used for financial forecasting, such as regression analysis, time-series analysis and simulation modeling. The specific approach used will depend on the type of data available, the complexity of the financial situation being analyzed, and the goals of the forecasting effort. We’ll explore these methods with some detail.
Financial Forecasts – What are the Options?
There are several methods for financial forecasting. I’m going to list the most common options and then dig deeper into the most popular, but please realize that one size doesn’t fit all, and the best method for your business will vary on several factors.
The most common methods are:
- Trend analysis: This method involves analyzing historical data to identify trends and patterns that can be used to forecast future performance. For example, you might look at sales trends over the past few years to estimate future sales growth.
- Regression analysis: Regression analysis involves using statistical techniques to identify the relationship between different variables. For example, you might use this to identify the relationship between sales and advertising spend, and then use that relationship to forecast future sales.
- Time-series analysis: This method involves analyzing historical data to identify patterns and trends over time. For example, you might use this analysis to identify seasonal trends in sales or revenue, and then forecast future performance.
- Scenario analysis: Scenario analysis involves developing different scenarios based on different assumptions about the future. For example, you might develop a “best-case” scenario, a “worst-case” scenario and a “most likely” scenario. You can then use these to forecast future performance.
- Expert opinion: This method involves soliciting the opinions of experts in a particular field. For example, you might ask industry experts to provide their forecasts for future market conditions or economic trends.
- Simulation: Simulation involves creating a computer model that can be used to simulate different scenarios and outcomes. For example, you might create a simulation model to forecast the financial impact of different strategic decisions.
These are just a few of the forecasting methods. The choice of method will depend on the specific needs and circumstances of your business or organization.
The accuracy of these methods depends on various factors, including data quality, assumptions and the complexity of the models used. There is no single method that is universally more accurate than the others. Each method has its strengths and weaknesses, and their accuracy can vary depending on the specific context and the data quality.
For example, trend analysis may be more accurate in industries where sales or revenue growth is relatively stable and predictable, while scenario analysis may be more appropriate in industries with a high degree of uncertainty and volatility. Similarly, expert opinion may be more useful in situations where the expertise of the individuals consulted is particularly relevant and valuable.
It’s often useful to use a combination of different methods to generate a range of forecasts and consider the potential outcomes from each method. This can help to mitigate the potential inaccuracies of any single method and provide a more comprehensive picture of the possible scenarios.
Let’s dig a bit deeper into two of the methods that I see businesses often using for forecasting – time-series analysis and regression analysis.
Time-series analysis is a statistical method to identify patterns and trends in historical data. It’s a powerful tool. The basic steps are as follow:
- First, gather the historical data you want to analyze. This data may include sales figures, revenue, expenses or any other financial metrics that you want to forecast. Hopefully, this data is highly dependable and resides in a modern financial management platform.
- Once you have the data, you need to clean it to ensure that it is accurate and reliable. This may involve removing any outliers or errors, and then adjust for any seasonality or trends that could skew the analysis. If your data is in a modern financial management platform, it should be exceptionally clean, but if you’re dependent on spreadsheets, this will slow the process down considerably.
- Plotting the data on a graph is a useful way to visualize any trends or patterns. This can help you identify any recurring patterns or trends that may be relevant to your forecast. Interactive data visualization tools make this step much easier.
- Calculate the mean, median and standard deviation of the data to gain a basic understanding of its distribution and variability. This information can help inform your forecast.
- Next, choose a time-series model. There are various models available, and the choice of model will depend on the nature of the data and the goals of the analysis. Some common models include moving averages, exponential smoothing, and autoregressive integrated moving average.
- Apply the chosen time-series model to the data to generate a forecast. This may involve extrapolating the trend or pattern observed in the historical data into the future or applying statistical techniques to identify any seasonal or cyclical patterns.
- Finally, evaluate the accuracy of the forecast by comparing it to actual data as it becomes available. This can help you refine the model and improve the accuracy of your forecasts over time.
Unlike time-series analysis, regression analysis is a statistical method that identifies the relationship between different variables to generate a forecast based on that relationship. Overall, regression analysis is an immensely powerful tool for financial forecasting. Its basic outline is as follows:
- The first step is to identify the variables that you want to analyze. This may include sales figures, revenue, expenses or any other financial metrics that you want to forecast. You will also need to identify any potential independent variables that may be related to the outcome variable you are trying to forecast, such as advertising spend or interest rates.
- Once you have identified the variables, you need to collect the historical data for each variable.
- Clean the data to ensure that it is accurate and dependable. This may involve removing any outliers or errors in the data, adjusting for any seasonality or trends that could skew the analysis.
- Now it’s time to choose a regression model. There are several types, including simple-linear, multiple-linear and polynomial. The choice of model will depend on the nature of the data and the relationship between the variables.
- Run the regression analysis using a statistical software package. The software will generate a mathematical equation that describes the relationship between the independent variable(s) and the dependent variable.
- Evaluate the results to determine the strength and significance of the relationship between the variables. This may involve calculating the R-squared value, which measures the proportion of the variation in the dependent variable that can be explained by the independent variable(s).
- Once you have identified the relationship between the variables, you can use the regression model to generate a forecast for the dependent variable based on the values of the independent variable(s).
- Finally, evaluate the accuracy of the forecast by comparing it to actual data as it becomes available. This can help you refine the model and improve the accuracy of your forecasts over time.
Whether you choose these methods or a combination of these and others, there are some important considerations. Overly optimistic or pessimistic assumptions can lead to unrealistic forecasts. It’s important to base your assumptions on data and research, and to be conservative in your estimates. As well, you’ll need to consider any seasonality or trends that may affect the variables being forecasted. Ignoring these factors can lead to inaccurate forecasts. External factors, such as changes in the economy, regulatory environment or consumer behavior, can have a significant impact on financial outcomes. It’s critical to consider these factors and incorporate them into the forecast.
Financial forecasts should be updated regularly to reflect changes in the business environment or any new information that becomes available. Failing to update the forecast can lead to inaccurate or outdated information. And while historical data is a vital input for financial forecasting, the future may not follow past patterns. It’s important to incorporate other inputs such as market research and expert opinions into the forecast.
Also, consider alternative scenarios that may affect the forecasted outcomes. This can help identify potential risks and opportunities to enable better decision-making. Lastly, using a range of forecasting methods will help you generate more accurate forecasts. Different methods may be more appropriate for different types of data or situations.
Financial Forecasting Benefits
Forecasting provides several benefits, including:
- Better decision making: Financial forecasting helps businesses make better-informed decisions by providing a clearer understanding of their financial situation and potential outcomes. Forecasting helps identify potential risks and opportunities, and assist with strategic planning, budgeting and resource allocation.
- Better financial management: Forecasting helps companies manage their finances more effectively by providing insights into cash flow, revenue, expenses and profitability. This facilitates financial planning and enables proactive decision-making.
- Better funding and investment decisions: Forecasting helps businesses attract funding and make investment decisions by providing a clearer picture of their financial performance and future potential. This can help with securing loans, attracting investors and making informed investment decisions.
- Better business performance evaluation: Financial forecasting is used to evaluate the business performance over time. By comparing forecasted outcomes with actual results, businesses can identify areas for improvement and adjust their strategies accordingly.
- Better accountability and transparency: Forecasting helps businesses demonstrate accountability and transparency by providing a clear picture of their financial situation and plans. This can be important for maintaining trust and credibility with stakeholders, including customers, employees, investors and regulators.
When to Make a Financial Forecast
Businesses should put together financial forecasts at regular intervals as part of the financial planning process. Forecasting is an ongoing activity and should be conducted on a regular basis to provide a continuous view of the business’s financial situation and to adjust its financial plans as needed. That said, there are some specific times when a business should look at producing a forecast:
- Businesses should create a forecast for the coming year at the beginning of each fiscal year. This forecast can help guide financial planning, budgeting and resource-allocation decisions.
- When considering a major investment, expansion or other significant business decision, it’s essential to conduct a forecast to assess the financial impact of the decision.
- Whenever a business is entering a new market or launching a new product, a forecast is needed to assess the potential revenue and profitability of the new venture.
- When seeking funding from investors, lenders or other sources, a forecast can demonstrate the business’s financial performance and potential for growth.
Even when there are no major changes or decisions to be made, companies should conduct regular forecasts on a quarterly or annual basis to assess financial performance, identify potential risks and opportunities and adjust financial plans as needed.
In summary, an accurate financial forecast will serve as the basis for budgeting decisions and provide a gauge for those making material financial decisions. Regardless of model, the foundation for accurate forecasts is reliable data. Your choice of where that data comes from can substantially affect the forecast and the performance of your business.